SQL Performance with Server-Side Flash Acceleration
There is one storage technology that is currently sweeping the IT industry and revolutionizing performance, and that is NAND flash, in the form of SSDs, EFDs, and PCIe devices. When it comes to SQL performance, we think the lyrics of the Queen song “Flash Gordon” are very appropriate (see Figure 6.38). I wonder if they could see the future of enterprise and web-scale data centers when they wrote that song? Either way, as the previous section illustrated with the discussion around SSD and EFD in your storage array (including All Flash Arrays), it liberates performance for SQL from the tyranny of slow spinning disks that may no longer be economic.

Figure 6.38 Flash acceleration and lyrics from the classic Queen song “Flash Gordon.”
But flash in an array has some limitations, and there is another location where we can use flash SSDs, EFDs, and PCIe that can greatly improve SQL performance, directly in the VMware ESXi servers hosting SQL. This is where server-side flash and associated acceleration solutions come in. Server-side flash when used as part of an IO acceleration solution can be thought of as cheap memory, rather than expensive disk. It is definitely cents per IOP and dollars per GB, but the returns on investment and performance can be substantial. Especially when it is not possible to add more RAM to the buffer cache, which would be the fastest possible storage from a performance perspective.
By using server-side flash acceleration, you can normally consolidate more SQL VMs per ESXi host, with less memory directly assigned to each SQL VM, and without sacrificing performance and user response times. Read or write IOs are offloaded to the local server flash device, and this acts as a very large cache. It can also greatly reduce the load on the back-end storage, which allows the array to improve its efficiency.
Because the flash devices are local to the server, the latencies can be microseconds (us) instead of milliseconds (ms) and eliminate some traffic that would normally have gone over the storage network. By reducing the storage IO latencies, not only are user response times improved, but overall server utilization is improved. You may see increased CPU utilization, as you are able to get more useful work done by reducing system bottlenecks.
In this section, we cover three different server-side flash acceleration solutions that are supported with VMware vSphere and can greatly improve the performance of your SQL databases. The solutions we cover are VMware vSphere Flash Read Cache (vFRC), which is included with vSphere 5.5, Fusion-io ioTurbine (IOT), and PernixData Flash Virtualization Platform (FVP). The first two solutions act as a read cache only, as all writes go directly to the backend storage while being cached and are therefore write through. PernixData FVP, on the other hand, offers a full write back cache, where both read IO and write IO can be accelerated.
VMware vSphere Flash Read Cache (vFRC)
vSphere 5.5 introduces vSphere Flash Read Cache, or vFRC, which is an infrastructure layer that aggregates flash devices into a unified flash resource pool. vFRC supports locally connected flash devices such as SAS/SATA SSDs and PCIe. The flash resource can be used to cache read IOs and is configured on a per-VMDK basis. The vFRC write policy is write through, which means that all writes go to persistent storage and are cached in vFRC simultaneously. To prevent pollution of the cache, large sequential writes are filtered out. Each VMDK flash resource allocation can be tuned based on the workload. For SQL, it’s recommended that data file VMDKs and Temp DB VMDKs be configured for vFRC when used, whereas transaction log will usually have little benefit.
Figure 6.39 shows a high-level overview of the VMware vSphere Flash Read Cache architecture.
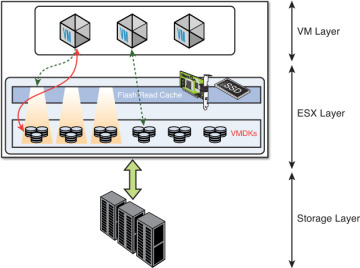
Figure 6.39 vFRC architecture overview.
The types of SQL workloads that will benefit from vFRC are read-dominated OLTP-type systems and read-dominated data warehouse queries. The ideal workload has a high repeated access of data—for example, 20% active working set that is referred to 80% of the time.
The major determinants of performance are the cache size, the cache block size, and the type of flash device used (SSD vs. PCIe). In terms of cache sizing, it is important to ensure that the cache is big enough to cover the active working set without being too big that you’re wasting the valuable flash resource. The cache block size should be equal to the dominant IO size of the VMDK; for SQL, this will be predominantly between 8KB and 64KB. If you are unsure of the main IO size for your database, you can use vscsiStats for a period of time to record the IO profile. To learn more about vscsiStats, see http://cormachogan.com/2013/07/10/getting-started-with-vscsistats/.
The type of flash device used will have an impact on the overall IOPS and latencies you can achieve. Although SATA and SAS SSDs are cheaper, they do not offer the same performance as PCIe. The right device for your environment will depend on your workload, performance, and budgetary requirements.
Having a cache block size that is too big can cause fragmentation in the cache and poor utilization. This may cause a substantial portion of the cache resource to be unutilized and therefore wasted. Figure 6.40 illustrates the impact of vFRC block fragmentation.
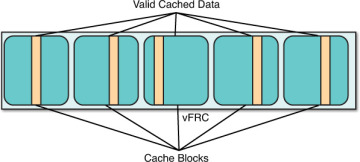
Figure 6.40 vFRC block fragmentation.
In Figure 6.40, the vFRC block is set to a much larger size than the predominant IO size—in this case, 128KB or 512KB versus the actual IO size of 8KB. As a result, a large proportion of the blocks configured is wasted.
The cache size and block size are manually set when you enable vFRC on a VM, and they can be changed at runtime without disruption. Having the cache too small will cause increased cache misses, and having it too big is not just wasteful, it will impact your vMotion times. By default, when vFRC is configured, the cache of a VM will be migrated when the VM is vMotioned. If it’s set too big, this will increase the vMotion times and network bandwidth requirements. You can, if desired, select the cache to be dropped during a vMotion, but this will have an impact on SQL performance when the VM reaches its destination while the cache is being populated again.
Fusion-io ioTurbine
ioTurbine is caching software from Fusion-io that leverages the Fusion-io ioMemory range of high-performance flash devices, such as the SLC- and MLC-based ioDrive and ioScale PCIe cards. ioTurbine creates a dynamic shared flash pool on each ESXi server that can be divided up between cache-enabled VMs based on proportional share algorithm. By default, each VM is assigned the same shares and thus get an equal proportion of the available flash cache resource pool.
Like VMware’s vFRC, ioTurbine is a read cache, and all writes are sent through to persistent storage while simultaneously being cached. Unlike vFRC, there are no manual parameters to set on a per-VM basis to size the cache or the blocks that are cached. This automatic and dynamic sizing of the flash cache of each VM is useful where you have lots of VMs that can benefit from caching or where you have flash devices of different sizes on different hosts. It reduces the management overhead.
Figure 6.41 displays a high-level overview of the ioTurbine architecture, including Fusion-io’s Virtual Storage Layer (VSL) driver. As of ioTurbine 2.1.3, which supports vSphere 5.5, the VSL SCSI driver is used by default instead of the VSL block driver. This can provide improved performance and better resiliency.
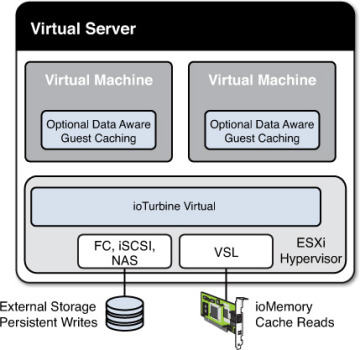
Figure 6.41 ioTurbine architecture overview.
In addition to being able to cache a VM, ioTurbine is capable of caching disks, files, and entire volumes. With the optional in-guest agent, the caching becomes data and application aware. This means particular files within the OS can be cached while others are filtered out. This is very useful for SQL where we only want the data files and Temp DB files cached while the transaction logs are not cached.
ioTurbine is fully compatible with VMware features such as DRS, HA, and vMotion. ioTurbine also works in environments where not all ESXi hosts contain a flash device, in which case the flash cache of a server would be set to 0.
In the example in Figure 6.42, if one of the VMs in the left ESXi host is migrated to the right ESXi host, all VMs will be allocated one third of the flash cache capacity of each host because there will be three cached VMs on each host.
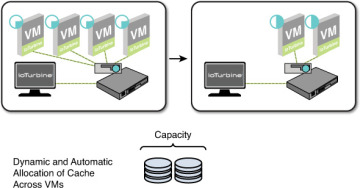
Figure 6.42 ioTurbine dynamic and automatic allocation of flash cache.
Table 6.19 was obtained from Fusion-io performance test results published at http://www.fusionio.com/blog/performance-of-a-virtualized-ms-sql-server-poor-ioturbine-to-the-rescue. The results demonstrated that by offloading reads to the ioTurbine flash cache, write performance also increased by just over 20%. This test was based on TPC-E workload. This demonstrates that read caching can also improve write performance to a certain extent.
Table 6.19 ioTurbine SQL Server Performance Example (TPC-E)
ioTurbine Off |
ioTurbine On |
Improvement |
|
Avg. Duration (us) |
146,861 |
29,800 |
400% |
Avg. CPU Time Consumed |
22 |
22 |
None |
Total Reads |
95,337,525 |
127,605,137 |
34% |
Total Writes |
34,901 |
43018 |
23% |
PernixData FVP
PernixData FVP is different from the other two solutions already discussed in that it aggregates server-side flash devices across an entire enterprise to create a scale-out data tier for the acceleration of primary storage. PernixData FVP optimizes both reads and writes at the host level, reducing application latency from milliseconds to microseconds. The write cache policy in this case can be write back, not just write through. When the write back cache policy is used, the writes are replicated simultaneously to an alternate host to ensure persistence and redundancy in the case of a flash device or host failure.
Application performance improvements are achieved completely independent of storage capacity. This gives virtual administrators greater control over how they manage application performance. Performance acceleration is possible in a seamless manner without requiring any changes to applications, workflows, or storage infrastructure.
Figure 6.43 shows a high-level overview of the PernixData Flash Virtualization Platform architecture.

Figure 6.43 PernixData FVP architecture overview.
The flash devices in each ESXi host are virtualized by FVP, abstracted and pooled across the entire flash cluster. As a result, you can have flash devices of differing types and sizes in different hosts. Ideally though, you will have a homogenous configuration to produce more uniform performance acceleration. Hosts that don’t have local flash devices can still participate in the flash cluster and benefit from read IO acceleration. This is termed a “non-uniform configuration,” when some hosts have local flash devices and some don’t.
In the case of a non-uniform flash cluster configuration, when a VM on a host without a flash device issues a read operation of data already present in the flash cluster, FVP will fetch the data from the previous source host and send it to the virtual machine. Because there is no local flash resource present, it cannot store it locally; however, FVP will continue to fetch data from the flash cluster to keep the latency to a minimum while reducing the overall stress and load on the storage array.
With PernixData FVP, it may be possible to delay the need for costly forklift upgrades of existing primary storage investments that have reached the end of their performance, well before the end of their capacity. As we’ve seen with our RAID calculations, this can be common for high-performance workloads. FVP can provide much more efficient use of the deployed capacity and may allow the breathing space required for you to determine the best next steps for your future storage and virtualization strategies.
The examples in Figures 6.44 and 6.45 show a SQL 2012 database driving around 7,000 IOPS consistently and the resulting latency both at the data store and at the VM level. The total effective latency is what the virtual machine sees, even though the data store itself is experiencing drastically higher latency. In this case, in spite the latency of the data store being upwards of 25ms, the SQL VM response times are less than 1ms.
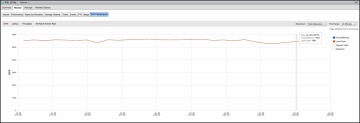
Figure 6.44 PernixData FVP acceleration for SQL Server 2012 IOPS.
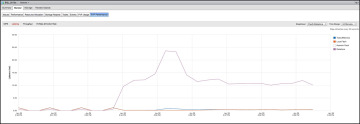
Figure 6.45 PernixData FVP acceleration for SQL Server 2012 latency.
When FVP cannot flush the uncommitted data to primary persistent storage fast enough—that is, when more hot data is coming in than there is flash space available—FVP will actively control the flow of the new data. This means that FVP will artificially increase the latency, ultimately controlling the rate at which the application can send, until the flash cluster has sufficient capacity and returns to normal. FVP does not transition to write through, even when it is under heavy load. Applications normally spike and are not continuously hammering the data path 100% all time, so FVP flow control helps smooth out the “spikey” times, while providing the most optimized performance possible.